Member Profiles: A Conversation with Lindsay Paterson
In the second of our series on member profiles, Centre for Statistics Co-Director Valeria Skafida interviews Lindsay Paterson, Professor Emeritus of Education Policy.
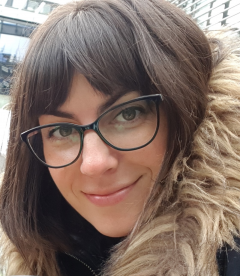
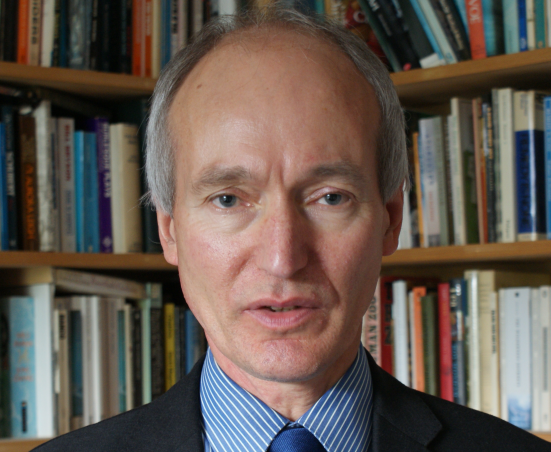
Valeria Skafida: We are here to talk about your career and your life, and the role of statistics within it. Let’s start with what could be considered a starting point: what drove you to enrol into a PhD in statistics at the University of Edinburgh?
Lindsay Paterson: The starting point is of course before then because I came from a maths degree. Because I went to a Scottish university in the 1970s, it was still possible to have a broad range of subjects. I studied various subjects but mainly maths and English, and so, by the time I got into second year, I had the option of doing honours English or honours maths, and I chose maths, essentially because I found it easier. There are few things more satisfying than getting a maths puzzle correct. It's a beguiling sense of perfection, that sense that you can actually get a right or wrong answer. When I got to the end of my four years as an undergraduate, I then was faced with the question of what to do next. And I then decided that spending my life enjoying solving mathematical puzzles or inventing them as a researcher was, after all, never going to be sufficiently satisfying for ever. So I decided that I wanted to do something useful, and at the time this felt like choosing between statistics or economics. I chose statistics for essentially the reason that the head of the maths department in Aberdeen at that time, Professor Edward Patterson (no relation to me), had a brother – Dr Desmond Patterson (later professor) – who was associated with the statistics department at Edinburgh University and who was looking for a PhD student to study a particular mathematical problem in designing experiments for agricultural science. So that's how I ended up here at Edinburgh – a mixture of random, chance networking and of a desire to do something useful with the mathematics that I'd enormously enjoyed but couldn't see myself spending 40 years doing.
From Statistics to Sociology of Education
VS: Your contributions to the sociology of education and education policy research are renowned. What drove you to this field? Do you feel that this was your calling or is this also a chance encounter?
LP: That is something I have asked myself about a lot because it's one of those things provoked by retiring, which I did last year in 2022. The opportunity to study for the PhD, which is a purely mathematical PhD, came up because of the potential application in agricultural science, but it wasn't an agricultural PhD. I knew absolutely nothing about botany, or about engineering, or about farm management. My PhD was therefore essentially a PhD in number theory. I've just read the marvellous book by Professor Jim al Khalili on Arabic science in the middle ages, and one of the things he talks about is the place of number theory in Arabic mathematics, building on Indian origins. My PhD was an opportunity to do mathematics of that fascinating kind. I was very fortunate that my PhD supervisor didn't care that I didn't know anything about agriculture, because I would then, in a postdoctoral opportunity, see how number theory could be applied to this field. That was the second step: I got what was in effect a postdoctoral fellowship writing computer programmes to apply the number theory that I had developed to make agricultural experiments more efficient.
After that, I went to a lectureship in statistics at Heriot-Watt University. Then, through random meetings, I got to know Dr (later Professor) Gillian Raab, who was looking for a spare statistician to help her and another statisticians in a project called the Edinburgh Lead Study. This was a large epidemiological study of the effects of environmental lead exposure on children's development – a very cross disciplinary committee that included educational psychologists and sociologists (as well as medics and environmental scientists). Through them I got to know the people in what was then the Centre for Educational Sociology. I first started working externally with them and then I got a job there. So that was all in a sense, random, but of course, there were other opportunities that I turned down. So I suppose the answer to your questions: Why education? Why sociology? … was that actually that combination inspired me in a way that, for example, other parts of epidemiology that I could have ended up in didn't to the same extent. So that then took me into educational sociology where I essentially have stayed ever since. That is despite my title saying “education policy”: in a democracy, every citizen is a policy-maker, and so the proper study of the policy process is fundamentally sociological and thus inescapably statistical.
Another way of putting the story is this. I had a degree in mathematics and a PhD in pure mathematics. From that I forced myself to learn statistics and, especially through teaching at Heriot-Watt, I learnt in a rudimentary sense most of the standard techniques. The language of mathematics and the language of statistics then enabled me to go into new fields. And thus the territory became something that I could choose. Having degrees in mathematics and statistics made me more employable. So I'm not under any illusion, I hope: the reason I got the series of jobs in social science was sort of through the back door, because quantitative expertise is in short supply in various social science fields. Thus I've been more employable than people that are far better than me but don't have the quantitative background. I have used statistics, not to become a statistician, but to do something in the world. And to go back further, I used my mathematics not in order to be a lifetime mathematician, but to learn statistics, and then in turn to do something about the world. Now, that's just one route, but it is, I would say, a really satisfying route.
So if you're a new graduate from undergraduate mathematics or if you're a new graduate from a PhD in statistics, following that route can be very fulfilling, but it does open up this dilemma. Do you continue to be primarily a mathematical statistician, whose main contribution to scholarly literature is solving mathematical problems? Or alternatively, do you embed yourself in sociology or epidemiology or some other discipline where people are using statistics and mathematics? When I was at Heriot-Watt in a statistics department I felt that the thing I was lacking was the richness of the embedded knowledge that working continuously everyday with sociologists or psychologists would give me. But after a few years of having embedded myself among sociologists, I then thought: “Oh, God, I'm missing the mathematicians! I missing that rigorous correction!” And although I continue to discuss things with mathematicians and statisticians, I miss that daily interaction. I think that's a perpetual dilemma. There are very few social scientific contexts that I know of that manage to maintain the two things at the same time, the embeddedness in a substantive discipline as well as the ready access to mathematical and computing expertise – computer science, or coding –that can make sure you're on the right track technically.
Activism and Policy Impact
VS: I see this desire, this drive to contribute to society. You have been very vocal activist in the Scottish education policy reform scene throughout your career. Yet, this kind of impact work requires a very different set of skills to the ones we are trained in as academics. What are the key insights that you wish to share about this experience and managing this parallel life?
LP: Yes, it is the same desire that took me away from pure maths to applied maths in the form of statistics and then from theoretical statistics to applied statistics, and from theoretical sociology to applied sociology. I think this type of work is an important thing that some academics should do, but I wouldn't for a second suggest that all academics should do it. I think any big university, like Edinburgh, ought to have a sufficient number of people engaged in the policy world – all kinds of policy, including for example, let's say, policy on vaccines or policy on agricultural science or policy on wave engineering; but that doesn't mean any individual academic ought to do that. I think in fact that any university that was only about applied ideas would not be a proper university.
So I'm totally parasitic in three ways: on pure maths, on pure statistics, and on pure sociology. I think one of the fantastic things about broad universities like Edinburgh – one of the things that makes this kind of university one of the most amazing institutions that human beings have created – is the capacity to exchange ideas completely freely without any fetters whatsoever on what is said. I feel very privileged to have enjoyed that freedom. I think that kind of debate and the resulting knowledge are crucial, whereas the applied knowledge is not crucial to a university. But, for me, a certain reservation about pure knowledge has always been a motivation. I couldn't be content with just writing theoretical texts about social mobility any more than I could have been content writing about the loglinear models that allow us to study social mobility. I think that the key thing here is applying all that stuff. In doing that, I am dependent on people developing the fundamental ideas that people like me can then purloin.
So what is it that the statistics and the mathematics bring to applied sociology? I think that that is something which is really deeply missing from public discourse – not just in Scotland or Britain, but actually very widely: anything more than a really quite elementary appreciation by our policymakers of the rigour of proper mathematical thinking. I do think mathematics provides the absolute model of rigorous human thinking, more rigorous than any other form of thinking that I have ever come across. And therefore, unless somebody has trained – that doesn't mean at an early age, by the way – but unless somebody has really got to grips with the rigour of mathematical thinking, then it doesn't seem to me that they are entitled to say that any other modes of thinking are in fact more rigorous. It's probably not controversial to say that to an audience of statisticians, but undoubtedly it's extremely controversial in the social science faculty at the university.
Reflections on Statistics
VS: And logic is built on maths, as I recall from studying the philosophy of logic?
LP: Indeed, and that reminds me that my PhD supervisor started me off on this too. I had asked for his recommendation of philosophy books to read, to which he suggested Karl Popper. I did, and immensely enjoyed it, above all because he is one of the few philosophers that scientists find useful. Popper’s is a system of formal logic (in The Logic of Scientific Discovery). Or you can read A. N. Whitehead and Bertrand Russell, who take hundreds of pages to get to the proof that one plus one equals two which is quite fun but also provides a model of logical rigour. I have to say that as an undergraduate, one of the most enjoyable courses I took was on mathematical logic. And one of the most satisfying proofs that I ever had to confine to memory for the purposes of examination was Gödel’s incompleteness theorem. That is a marvel like the Sistine Chapel or the Hagia Sophia, or the Taj Mahal. Theorems like that are absolutely wonderful creations of the human mind. So, the key thing here is, that I have tried, in a very much more mundane realm but being inspired by these great heights, to bring a rigour of mathematical thinking to public discourse, whether that's with politicians or journalists, or in my case with audiences of school teachers or in other parts of higher education.
This kind of thing is very much in short supply. The UK Prime Minister, Rishi Sunak, has recently said that everybody should study mathematics to age 18. I have a lot of sympathy for that (though I think he was completely naive about the possibility of it ever happening). If we think it's possible to be an educated human being and not understand mathematics to roughly the equivalent of upper-secondary schooling, then we are not really as well educated in our mathematical thinking as we are in our linguistic or philosophical or historical thinking. So I have tried to find ways in which I can translate my academic education into forms that are socially useful, both in the sense of offering comments on policy, but also I hope, in helping to educate the civic society in the necessity of thinking of a policy in rigorous ways.
VS: Has your understanding of the possibilities that statistical methods (broadly defined) can offer, changed or evolved at all throughout your career by having worked in and applied these methods in different disciplinary environments?
LP: Yes, absolutely. So you start off as mathematician thinking that you can represent the world in mathematical ways. I remember early on reading the wonderful writer, D’Arcy Wentworth Thompson, Professor of Natural History at what was then the University College of Dundee. He wrote a beautiful book in 1917 called On Growth and Form. It's about the essentially mathematical nature of the natural world. This was a pioneering book because it appeared just at the point at which the Mendelian tradition in genetics was coming together with the Darwinian one. So it's at the very beginning of modern genetics. He's already talking about the question of how one side of a leaf knows that it has to be symmetrical with the other side. We now know how that happens, but he wrote about it in purely mathematical terms. So I kind of grew up with this fanciful notion that the world could be described in mathematical terms.
But what I have come to learn, especially through working in social science, is that that's not what statistics is trying to do. It is a fallacy to think that what we're doing with statistics most of the time is actually representing real world processes. What we're doing, even in the most elaborate, sophisticated model, is simply summarising a description. We’re producing a description of reality not a model of reality. We use the word “model”, but it’s not really a model. It's a description. The Stone–Weierstrass theorem – another of these unforgettable beauties of pure mathematics – says that for any reasonably well-behaved function, you can approximate it by a polynomial of high enough degree. Now that’s quite mind blowing, because it says that no matter how complex the true reality is, you can essentially describe it with something as simple as a polynomial. We don’t have to worry about our models being an accurate representation of the real underlying processes. All we need to do is find a sufficiently succinct description of reality that for the time being is enough. This point has reminded me of medieval nominalism, the forerunners of modern science. Fourteenth-century thinkers such as Nicholas Oresme in Paris, and William of Ockham in Oxford, argued that the appropriate use of mathematics is as a way of summarising our sense-impressions, not as a model of the world in the manner that derives ultimately from Plato. (This scepticism, too – like number theory – was a legacy of Arabic thinking.) Freeing empirical thought from the need to speculate about ultimate reality, it prepared the ground for the development of modern science. Statistical analysis is the heir of this tradition.
But what’s descriptively sufficient is different in microbiology to what it is in, let's say, economics at an aggregate macro level. The point is that then what is sufficient depends on the substantive discipline. What we understand by an acceptable error in particle physics is tiny compared with when there is an error in sociology. What is an acceptable degree of significance in the recent developments of genetics – where you have massive datasets and you are studying tiny locations on the genome – has to be enormously more rigorous than would be required in the test of effective methods of teaching children to read. No matter what the field, our statistical calculations or models are finding a succinct way of summarising the complexity of our statistical data. They are not actual representations of the real world. My view of that has changed fundamentally over the over the decades.
Recent Research on the Evolution of Scottish Education
VS: Thank you Lindsay. Now, you've completed a three year research project, funded by the Leverhulme Trust called Education and Society in Scotland. It would be nice if you could tell us a little bit about this project and what the findings were.
LP: As a preliminary to doing that, there is something I forgot to say about applying things to policy. All policy is local, especially democratic policy. Democracy is the most parochial form of government that has ever been invented. It punctures the potential grandiosity of politicians all the time because they have to always come back to the electorate to seek their votes. So, as a researcher, even if you're working with a global organisation – such as, in my field, UNESCO or the OECD – you're only pretending that you're working with policy unless you translate from the global level down through national governments, usually also, in most countries, to regional governments, and eventually to local government in order to get to the classroom where real education happens. On the other hand, the ideal of academic life is not local, it's universal. It's scholars all over the world. We talk to each other all over the world. And we talk to each other through histories and you learn from things that were written two and a half thousand years ago, 1000 years or 200 years ago, not because you would think the same as them, but because sometimes they think differently, and that forces you to think in new kinds of ways. You have to transcend the local whether of time or of place, unlike democratic policy makers.
So to keep on engaging with policy you have to keep on engaging with the local and the present, but you do that in an academic context that is neither. Now there are two ways of resolving this potential tension. One is by comparing the same place across time; the other is by comparing between different countries. I made the choice long ago to engage in greater and greater depth with a specific country over quite a long period of time, a century and more. That's how I ended up with a project from Leverhulme, which is about a specific place which happens to be Scotland because there was an excellent series of school leavers surveys which are a great resource for doing this kind of historical comparison, linking the local to the universal ideas that have their origin in sociology and other disciplines.
VS: I've seen extracts from this project and presentations from it. What was the most challenging obstacle that you had to overcome in the running of the project?
LP: Yeah, well, the first thing was assembling the data from all the different surveys. There were around 150 different databases, with data from around a quarter of a million respondents stretching back for three quarters of a century. And of course, what you've got to do then is put the data into a form that can be analysed. And this is a point that we say over and over again to students doing statistical work: the results you see in a paper are smaller than the tip of an iceberg. And if you start running a model before several months of sorting out your data, you're probably doing it prematurely. Leverhulme were generous in letting me spend six to nine months just sorting the data out. That's the biggest challenge, and it was quite mind numbingly boring most of the time, it has to be said. But unless you do that, and if you jump in prematurely into statistical models, you're almost certainly going to be wrong, because you will miss important control variables. You will miss some of the ways, for example, in which ostensibly similar classificatory categories have actually changed over time. So what you think is the same label is not the same label as it was before. Then you have to decide which of these changes make a fundamental difference, and which may be subsumed into random error. These are big challenges, and the best I can hope is that the ways I have tried to resolve them provide an adequate approximation to the truth, no more than that. (The final product from this project is a book published by Edinburgh University Press, the electronic version of which is available free from the publisher’s website.)
Cherished memories and tips for budding statisticians
VS: We are coming to the last few light-hearted questions. Has any other statistician ever found mistakes in your statistical work?
LP: Yes, absolutely. Well, there are two different kinds of mistakes. An example of the first is in one of the big questions in the study of education: whether there is a causal connection between people becoming more educated, and people holding more liberal, tolerant views. Following in the footsteps of many researchers, I've done a little bit of work on this, using the British birth-cohort studies to see if acquiring more education does tend to shift people in a more liberal direction. And I’ve suggested in the conclusions, tentatively, that there's some evidence of that. However, in a very recent paper, Dr Elizabeth Simon has said these kinds of analyses, including my own, are insufficiently rigorous and do not have enough controls for the possible effects of early socialising. The main alternative explanation is a selection bias where the people who go into advanced education tend to come from the kind of social backgrounds already making them liberal. Dr Simon uses a very sophisticated design, essentially sibling controls, which shows that a lot of the explanation is in fact family socialisation. One should always welcome that kind of critique because it's done in a constructive manner, and will stimulate further research. (Simon’s paper in 2022 is ‘Demystifying the link between higher education and liberal values’, British Journal of Sociology, 73(5), pp.967-984.)
My second example is a quite different kind of error, one that provokes existential angst among anyone whose work depends on technical accuracy. I was at an early meeting when I was working on the Edinburgh Lead study. I had been assigned the task of analysing the lead content of children's teeth. (The children we studied were about the age of 8 or 9, and so their teeth were falling out naturally.) It was my earliest acquaintance with multi-level modelling, because you have within-mouth and between-child variation: there is far less residual variation in the lead levels between the teeth within children's mouths than there is between children. But this was way back in 1987, well before MLWin or R or Stata. It used the now defunct medical programme called BMDP. The software had the basic algorithm but you had to programme its application by hand. I had chosen to present the results in the form of predicted values, but what I forgot to do before the meeting was to include the intercept of the regression. So I presented these tables to this cross-disciplinary committee showing the estimated values of the level of lead in children's teeth. One very polite, wise professor said, “If this is correct Lindsay, these children would all be dead.” The intercept was negative, and so not adding it showed values of lead that would be poisonous. Now that was embarrassing. I hope I learned my lesson, which is that one should always cast a common-sense eye over the results. Does this look plausible? And in deriving the right common-sense instinct, it is crucial to pay attention to the substantive discipline. I try to force myself to run that common-sense eye across the data, and I hope it was that embarrassing moment when I had to report my data to that committee that led me to do that.
VS: Do you suffer from imposter syndrome?
LP: I think that suffering from it is a job qualification for being a decent scholar. If we don't doubt our capacity all the time, then we are becoming complacent and arrogant and not being open to correction. The reverse of that is that when a way in which your work could be improved is pointed out to you, it's not the end of the world. Because the flip side of the imposter syndrome is that when you're told that what you've done is not perfect, it's devastating and you go away and bury your head in the sand. In truth, neither of these reactions, neither the arrogant reaction nor the self-disparaging reaction, are really very sensible. The sensible scientific reaction, if somebody says something could be approved on what you've done, is to welcome that.
VS: Are there any key bits of advice you would offer to current PhD students using statistics in their studies?
LP: It would be different for two kinds of PhD students. Let me talk first about the PhD student who is like me, doing a PhD in broadly the context of mathematical statistics. That doesn't mean you have to have an undergraduate degree in maths but you've broadly spent your undergraduate years as a mathematician and you're doing a PhD in the mathematical context. Unless you want to be a mathematician, and if you want to use your statistics, do the best you can to start working in cross-disciplinary teams. You cannot be a plausible applied statistician without working with the experts in the substantive areas, whether these are biologists or chemists or sociologists or economists or psychologists etc. You've got to establish these networks, and I think that's the most challenging thing to do. You've got to find people that you will work with collegially and that's very difficult. The best way to do it is through finding established statisticians within your department, who are already working with these other networks which you then latch on to. The sociologists or the botanist or others who generate the data will be delighted if you come along and offer to do the analysis for them, but you've got to do that because otherwise all you're doing is playing the numbers. And that's not the real world.
The second group is of students who are doing primarily quantitative PhDs in a social scientific discipline, which is kind of where I am now and were a lot of my own PhD supervision has been. Here I think the key thing is to keep in touch with the mathematics of it. So it's like the opposite problem. Of course, mathematics these days includes computer coding. If you were to ask me, where do I use my maths most? It's not actually in the models themselves, because most of that has been programmed by somebody. Unlike when I was doing multi-level modelling 30 years ago, there are programmes to do all of that now. But writing coherent computer code for each new applied analysis is a form of mathematics, and coding is even more needed now than before because using other people’s clever algorithms needs coding to apply them. All the time, when I’m doing that, I think: this is like doing algebra. So as a social scientist doing quantitative work, you've got to be in touch with the most up to date mathematical thinking, including up to date coding thinking. That's why the Q-Step initiative has been so important because it has attempted to put quantitative undergraduates in touch with advanced mathematical coding and thinking. The more of that the better.
VS: Okay, I think I have my final question. Now that you’ve retired, what is your most treasured memory or experience in academia?
LP: I've been thinking of that partly because you alerted me to that question in advance, but partly also because it’s the sort of question that retiring tends to provoke. The first one that comes to mind was thus the extraordinarily generous send-off that I was given upon my retirement in 2022 by colleagues in the Social Policy department and from elsewhere in the university That will always stick with me as a lovely afternoon and evening. But of course, there are others as well. There was when I was elected as a fellow of the British Academy, and going to London for the presentation. You know, there is something overwhelming about feeling you belong to that grand British Academy building, just off the Mall, at the centre of one of the world’s great cities. But actually, if I'm really being honest, the satisfaction from both these examples depend on one’s vanity, which is always a dangerous temptation for an academic (which can be such a solipsistic profession). The thing that really satisfies me in a more self-less way is solving puzzles, solving problems. The satisfying thing is when you've got a puzzle, whether it's a mathematical puzzle or a social scientific puzzle. It is important you have thought about it, you've read about it, you've discussed it with other people, you've listened to what they've had to say. And then you try it out and you try to develop some sort of coherent answer. It is only ever a provisional answer, and can always be improved, but even a partial answer is what ultimately makes the life of the mind so deeply satisfying.
But here there is a danger. If you come from a mathematical background there is the sense of satisfaction and fulfilment that I mentioned at the beginning – of getting the right answer to a mathematical puzzle. The danger is to think that, in the social world of human beings, there is a right answer. If you think that you've produced the explanation of educational failure, for example, or the explanation of social inequality, then you're wrong. Because there isn't a complete explanation. So, being satisfied with an answer is itself a bit dangerous, and actually trying to be permanently dissatisfied should maybe be the aspiration of any academic.
VS: Thank you Lindsay, this has been very, very enjoyable. Thank you for opening up, I hope readers take inspiration from this interview. I certainly have!
Acknowledgements
Special thanks go to Ms Zain Kurdi for the meticulous transcription of the original interview.