Interfaces Between Statistics, Machine Learning and AI
The Centre for Statistics and the Bayes Centre were delighted to present 'Interfaces between Statistics, Machine Learning and AI'. The research day brought together researchers and practitioners working in the related fields of statistics, machine learning and artificial intelligence.
The day featured four invited talks and a poster session to share recent advances and catch up with colleagues. The event took place online.
Invited Talks:
Kobi Gal, School of Informatics
AI for Online Collaborative Group Learning
Collaborative student learning has been shown to lead to significant academic benefits among students, and to improved social skills that are critical for the workforce, such as communication and teamwork. However, these benefits were limited to small face-to-face groups and required the support of human experts who actively monitored and guided the group’s learning.
Technological advances now enable globally dispersed teams to collaborate online, from Q&A forums to virtual laboratories. Augmenting these settings with AI technology can scale up the benefits of collaborative group learning to online groups.
I describe challenges to AI research for supporting this new type of online teamwork, as well as opportunities for combining AI and ML towards supporting students' learning and teachers’ understanding of how students learn.
Peggy Series, School of Informatics
Bayesian Approaches to Understanding Mental Illness
A growing idea in computational neuroscience is that perception and cognition can be described in terms of predictive processing or Bayesian inference: the nervous system would maintain and update internal probabilistic models that serve to interpret the world and guide our actions. This approach is increasingly recognised to also be of interest to Psychiatry. Mental illness could correspond to the brain trying to interpret the world through distorted internal models, or incorrectly combining such internal models with incoming sensory information.
I describe work pursued in my lab that aims at uncovering such internal models, using behavioural experiments and computational methods. In health, we are particularly interested in clarifying how prior beliefs affect perception and decision-making, how long they take to build up or be unlearned, how complex they can be, and how they can inform us on the type of computations and learning that the brain performs. In mental illness, we are interested in understanding whether/how the machinery of probabilistic inference could be impaired, and/or relies on the use of distorted priors. I also introduce the emerging field of Computational Psychiatry and describe recent results relevant to the study of Schizophrenia and Autism.
Victor Elvira, School of Mathematics
Graph discovery and Bayesian filtering in state-space models
Modeling and inference in multivariate time series is central in statistics, signal processing, and machine learning, with applications in social network analysis, biomedical, and finance, to name a few. The linear-Gaussian state-space model is a common way to describe a time series through the evolution of a hidden state, with the advantage of presenting a simple inference procedure due to the celebrated Kalman filter.
A fundamental question when analyzing multivariate sequences is the search for relationships between their entries (or the modeled hidden states), especially when the inherent structure is a directed (causal) graph. In such context, graphical modeling combined with parsimony constraints allows to limit the proliferation of parameters and enables a compact data representation which is easier to interpret. We propose a novel expectation-maximization algorithm for estimating the linear matrix operator in the state equation of a linear-Gaussian state-space model.
Posters:
Publicly Viewable Posters
Anwar Alabdulathem: Tail Index Regression-Adjusted Functional Covariate
Lucía Bautista Bárcena: Statistical properties of the solutions to the stochastic Green function
Benjamin Cox: Parameter Estimation in Sparse Linear-Gaussian State-Space Models via Reversible Jump Markov Chain Monte Carlo
Thomas Fletcher: Inferential Data Modelling in a Query-Answering System
Sponsored by:

Hosted by:
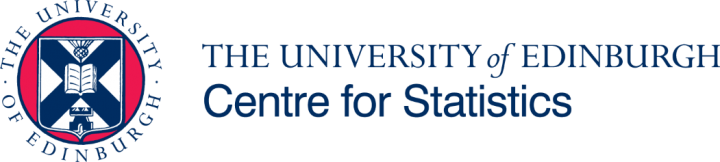
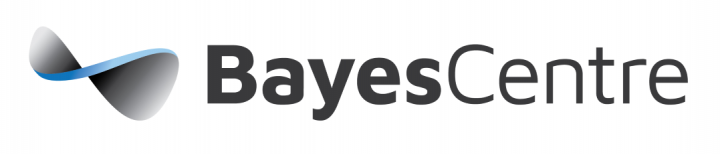
Interfaces Between Statistics, Machine Learning and AI
Online