Buzzwords unwrapped: Artificial Intelligence and Digital Twin
Prof. Chris Dent kicks off a series in which he "unwraps" current prominent "buzzwords"
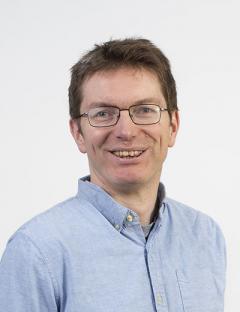
This is the first of an occasional series in which current prominent terms are unwrapped. Sometimes it is the case that there is a precise definition to be found which resolves all confusion over the meaning of such terms. More commonly however, it is not possible to identify a single definition on which everyone can agree, and the alternative to provide an explanation of the issues in play – which this article seeks to do. When using terms such as “AI” and “Digital Twin” which do not have settled meanings, it is usually helpful to explain what you mean, and if someone else uses such terms to ask them what they mean. Assuming people have a common understanding without checking is likely to prove a recipe for trouble.
This article surveys the range of meanings that the two terms can take, and provides a brief discussion of the issues underlying these ranges of different meanings. Understanding these is perhaps the most important step in using and interpreting the terms with confidence, as the terms themselves are in the end just words, whereas the underlying issues are the real substance.
Artificial Intelligence (AI)
AI is a very long standing concept[1], but we are very far from having a single agreed understanding of what it means. In my view, definitions fall into three broad classes:
- “Artificial General Intelligence”, or the “Turing Test”. AGI means replicating general human intelligence – the Turing Test refers to communication between a human and another entity, where the human has to work out whether they are communicating with a machine and another human; a computer passing the Turing Test would mean the computer being indistinguishable by other humans from a human interlocutor. I shall not discuss this much, as this is almost never the meaning given to “AI” in fields related to my own area of work, where an “AI” approach is typically directed at a single specific task.
- Methodology-driven definitions. This refers to definitions of AI in terms of certain classes of methodology – usually methods in which limited model structure assumptions are made, the idea being that the method “learns” from data, such as artificial neural networks (ANN), deep learning, reinforcement learning, or the broad group of approaches under “machine learning”.
- Application-driven definitions. These are definitions independent of a particular technology, such as referring to automated decision support/decision making, or carrying out tasks which would usually be deemed to require “intelligence” if a human were to carry them out[2].
My own personal inclination as an applied researcher is to use what I call above an application-driven definition, as this explicitly acknowledges the truism that a method should be chosen to suit the question, rather than the “AI” person having a hammer and going around looking for nails (or, worse, screws).
I once ad-libbed after a talk, when asked about relevance of AI methods in energy systems, that “the problem with Artificial Intelligence methods is that too often they are used with genuine stupidity”. At the time this was intended just to be a slightly crass joke, but I realised that it does convey a very important point. Mathematical techniques are not magic, in that they cannot do more on an applied question than the sum of the modelling and the data. However sometimes, perhaps because the term AI itself is very suggestive (as is ANN), methods under my “definition 2” have an image of capabilities that go well beyond the reality. In particular, approaches which make relatively little in the way of model structure assumptions tend to require extremely large amounts of data to produce good outcomes.
I certainly do not claim that data-driven methods such as ANNs and deep learning are not of very considerable value, and indeed they have led to major advances in recent years – a very prominent illustration is the phenomenal prowess of modern computer board game players[3], and there are many more across scientific, business, media and other sectors. It is simply the case that data-driven methods require a lot of data; one reason why such methods have been so successful in board games is that the number of games available for algorithm training is limited only by the computing resource available to produce example games through computer opponents playing each other. An authoritative discussion of issues in the development of AI and machine learning methods may be found in a talk by David Dunson of Duke University during a visiting period at Edinburgh University in 2018[4].
As well as limited volumes of data, there may be other reasons why one wishes to add modelling assumptions, for instance in many engineering applications it is natural to use physical models of the properties of the system to control or optimize its behaviour – this would fall under the title of AI in my third class of definitions. An extreme example of limited data occurs in most capital planning decision analysis questions, where there are no directly relevant measured (as opposed to modelled) data at all because the future has not yet happened.
Thus to summarise, in any application I simply advise choosing a modelling approach which is well suited to the properties of that application – or in the English vernacular ‘horses for courses[5]’.
Digital Twin (DT)
This is a much more recent term – indeed despite becoming commonplace in engineering modelling in the last few years it is not yet in the Oxford English Dictionary. An early use of the term is by Grieves in 2002[6], who gave the definition:
“The Digital Twin is the information construct of the Physical Twin. The intent of the Digital Twin is that it can provide the same or better information than could be obtained by being in physical possession of the Physical Twin. The key assumption is that the type, granularity, and amount of information contained in the Digital Twin is driven by use cases.”
Similarly, in its Gemini Principles[7] report, the UK national Digital Twin activity at the Centre for Digital Built Britain (CDBB) provides the following:
“Digital twins are realistic digital representations of physical things. They unlock value by enabling improved insights that support better decisions, leading to better outcomes in the physical world.”
The Gemini Principles go on to say that “What distinguishes a digital twin from any other digital model is its connection to the physical twin. Based on data from the physical asset or system, a digital twin unlocks value principally by supporting improved decision making, which creates the opportunity for positive feedback into the physical twin.” Additionally, IBM provide a helpful explanation and series of examples from their industrial perspective[8].
There is, however, no universally agreed definition[9]. Aspects commonly appearing in definitions, as in those quoted above, include that a DT is a high-fidelity representation of a real world object; that it should support improved decision-making; and that there should be feedback between the DT and the real world analogue.
It is also important to acknowledge that DT is not an entirely new concept, even though the widespread use of the term is recent. There are relevant recent developments, such as in data platforms and simply the widespread ability of large scale computing and data storage, which bring genuinely new capabilities in this area. However models which might now fall under the banner of “DT” have been used for a very long time in engineering system operation, for instance in electricity control centres, and uses in capital planning should certainly be seen as part of the broader and longer standing field of decision analysis or decision support.
One pitfall to avoid is over-emphasising the role of very detailed modelling across all areas of DT application. Very high fidelity models have a clear role e.g. in engineering design, and in optimizing/controlling system operation. However, in capital planning, and in strategic policy decisions, there is a different trade-off between fidelity (and thus computational expense) of modelling and the need to explore broadly the range of possible futures against which decisions are taken – the key point being that studies should be driven by the ultimate aim of supporting taking good decisions. In extremis, attempts at high fidelity modelling where the nature of future technology is very uncertain can become synonymous with “making stuff up”. These issues are well understood by both Grieves and by CDBB.
The notion of feedback between the DT and the real world system also varies greatly between use cases. For instance, where a model is used to support operation of an engineering system, a true closed loop picture - with information flows in both directions - is natural. However, in capital planning, it may take years to implement decisions, and then further years to assess the consequences, meaning that while feedback may occur eventually the decision analysis is really done in open loop.
Thus I return to a similar conclusion to that in my discussion of AI, which is well summarised by the vernacular “horses for courses”. Additionally, with “digital twin”, it is particularly important to remember that the emerging DT agenda sits in a broader and longer standing field of knowledge, and that not everyone who can contribute to discussions around “DT” will self-identify as being a DT expert.
References:
[1] The Oxford English Dictionary’s first example of use of ‘Artificial Intelligence’ is from 1955; the OED also states that Turing first proposed his Test in 1950.
[2] For instance, from the Hall-Pesenti Report on Growing the AI Industry in the UK: "Artificial Intelligence (AI) describes a set of advanced general purpose digital technologies that enable machines to do highly complex tasks effectively." The Engineering and Physical Science Research Council uses this description: "Artificial Intelligence technologies aim to reproduce or surpass abilities (in computational systems) that would require 'intelligence' if humans were to perform them." And from the Government Office for AI: "AI can be defined as the use of digital technology to create systems capable of performing tasks commonly thought to require intelligence."
[3] See for example https://deepmind.com/blog/article/alphazero-shedding-new-light-grand-games-chess-shogi-and-go
[4] https://www.ed.ac.uk/informatics/news-events/lectures/david-dunson-machine-learning-scientific-inference
[5] First recorded use in the OED is 1898, see https://www.oed.com/view/Entry/88583?redirectedFrom=horses+for+courses#eid1289693
[6] Grieves, M., Virtually Intelligent Product Systems: Digital and Physical Twins, in Complex Systems Engineering: Theory and Practice, S. Flumerfelt, et al., Editors. 2019, American Institute of Aeronautics and Astronautics. p. 175-200.
[8] See https://www.ibm.com/blogs/internet-of-things/iot-cheat-sheet-digital-twin/. Most examples available online are either too starry eyed about the term “digital twin”, are essentially sales pitches, or are too technically detailed to accompany this kind of piece. I think this IBM article strikes a good balance.
[9] Somewhat annoyingly, given its lack of respectability as a reference, the best concise collation of definitions I can find is in the Wikipedia article at https://en.wikipedia.org/wiki/Digital_twin#Definitions. A more scholarly survey of definitions and discussions of the term may be found in D. Jones, C. Snider, A. Nassehi, J. Yon and B. Hicks, Characterising the Digital Twin: A systematic literature review, CIRP Journal of Manufacturing Science and Technology, Volume 29, Part A, 2020, pp 36-52.
Chris Dent is Professor of Industrial Mathematics; Director of the Statistical Consulting Unit at the University of Edinburgh; and a Turing Fellow at the Alan Turing Institute.