CfS Annual Conference 2022
The Centre for Statistics Annual Conference brings together researchers working with data from across the University of Edinburgh and Associated Institutions.
This year the conference featured a number of talks from invited speakers on 14th June 2022.
All talks were recorded and are available to watch on Media Hopper!
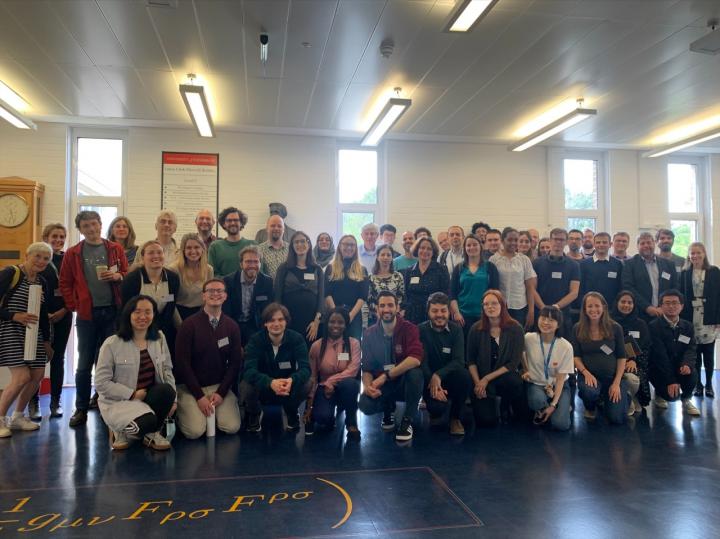
Organizing committee: Sara Wade, Cecilia Balocchi, Victor Elvira, Ozan Evkaya, and Nicola Branchini (PhD representative).
Invited talks feature speakers from the University of Edinburgh, across 8 Schools and Institutes, including the School of Social and Political Sciences, School of Informatics, Institute for Astronomy, School of Mathematics, Institute of Genetics and Cancer, Centre for Clinical Brain Sciences, School of Geosciences, and School of Engineering.
Contributed posters feature presenters from the University of Edinburgh across Schools and Institutes, including the School of Mathematics, MRC Institute of Genetics and Molecular Medicine, School of Geosciences, School of Health in Social Science, Institute of Genetics and Cancer and The Roslin Institute.
The full programme includes details of the poster session and a full list of participants.
Programme
09:00-09:30: Arrival w coffee/tea
09:30-09:45: Welcome (Victor Elvira, Director of Centre for Statistics)
09:45-10:15: Valeria Skafida (Social Sciences)
10:15-10:45: Diego Oyarzun (Informatics)
10:45-11:15: Coffee
11:15-11:45: Joe Zuntz (Astrophysics)
11:45-12:15: Simon Wood (Statistics)
12:15-14:15: Posters and Lunch
14:15-14:45: Riccardo Marioni (Centre for Genomic and Experimental Medicine)
14:45-15:15: Sotirios Tsaftaris (Engineering)
15:15-15:45: Coffee
15:45-16:15: Steven Hancock (Geosciences)
16:15-16:45: Maria Valdes Hernandez (Edinburgh Imaging/Clinical Brain Sciences)
16:45-17:00: Closing
Titles and abstracts
Valeria Skafida: Growing up with domestic abuse: insights from a Scottish birth cohort study and ethical reflections
Using a Scottish longitudinal birth cohort study, this talk showcases some recent research on domestic abuse prevalence, and outcomes for children living with domestic abuse. We will look at the extent to which experiences of abuse among mothers of young children are socially stratified and at how living with domestic abuse relates to children being victims of violence themselves. We also explore whether there are protective factors which can shield a child from the detrimental effects of domestic abuse. Finally, delving into more ethical matters, this talk will question what researchers can and what they should do when dealing with missing data in surveys covering sensitive topics such as this one.
Diego Oyarzún: Computational methods for biotechnology and biomedicine
In this talk I will give an overview of our work at the Biomolecular Control Group. Our team works on computational methods to study molecular networks in living cells. We use a mix of mathematics and computation to understand the inner workings of natural systems, as well as to design new biological circuits for biotechnology. We employ a wide range of methods for mechanistic modelling (optimal control, nonlinear dynamics, stochastic analysis), as well as data-driven models with various flavours of applied machine learning. Large parts of our work are in collaboration with wetlabs in the UK and abroad. I will particularly focus on two data-driven projects on a) machine learning for drug discovery, and b) genotype-phenotype prediction for biotechnology, and highlight the various data and statistical challenges that arise in them.
Joe Zuntz: Statistical Challenges for Upcoming Cosmology Surveys
Upcoming astronomical surveys like the Rubin Observatory and Euclid space probe will map galaxy locations and gravitational distortion across a large fraction of the observable Universe. Processing and analysing data from these surveys requires a wide variety of statistical techniques, from classical inference fo final science exploitation to the latest machine learning methods to characterise our data. I will give a short overview of some of the more interesting statistical challenges in the field and the methods being used to address them.
Simon Wood: Functional inference and Covid dynamics
Statistical methods for inferring functions have been well studied in statistics for half a century, but, unlike other statistical methods, do not seem to have been widely adopted in epidemiological modelling. This talk will illustrate how inference about incidence rates and the pathogen reproductive number, R, can be accomplished using relatively standard statistical functional inference methods. This includes cases in which the function of interest is embedded in a complex disease transmission model. Applying such methods to health service and daily mortality rate data indicated that UK Covid daily new infections were in decline, and R<1, some time before each national lockdown. These results were first available in April 2020 and were confirmed by subsequent reconstructions from randomized surveillance sampling studies.
Riccardo Marioni: How much about human health can we learn from a single blood test?
We know that both genes and the environment can influence our risk of developing diseases as we age. Whereas our genetics are fixed, our environment and lifestyle can be altered. One way we can track the influence of the environment is by studying chemical additions to our DNA that turn genes on and off. These additions, termed epigenetic modifications, can help us objectively measure factors such as alcohol consumption and smoking behaviour and build a picture of someone’s overall health. Using data from the Generation Scotland study, I will show how these patterns can help to improve prediction of disease, leading to healthier ageing.
Sotirios Tsaftaris: The pursuit of generalisation with real data
Modern machine learning has shown tremendous potential in a variety of domains including healthcare. For example, the interpretation of radiological images has seen considerable growth thanks to AI.
No matter the domain the holy grail is to devise models that can generalise: i.e. perform well on data beyond the training set. However spurious correlations exist in the datasets that we train with. Some may be frequent and omnipresent and some less so. And in some cases their existence is not readily visible or identifiable.
I will review recent theory inspired by a causal view to machine learning that can formalise spurious correlations and generalisation. I will then proceed to discuss several approaches from our team that can detect if models are susceptible to rare spurious correlations in real data, can build models that are invariant to correlations (e.g. scanner differences in medical images or differences between populations) thanks to disentangled representations, and can generate counterfactual data as means to correct for spurious correlations. I will conclude with limitations to hopefully inspire new solutions that traverse the world of causality, learning representations, and privacy/fairness. ( All the work presented is joint with students, postdocs, collaborators and other members of VIOS https://vios.science )
Steven Hancock: Lidar signal processing to increase the coverage and accuracy of global vegetation maps
Satellite lidar is the only way to directly measure tree height and canopy cover profile from space. These measurements enable the accurate modelling of essential climate variables like biomass and biodiversity. Lidar measurements require the ground to be accurately detected which requires there to be sufficient lidar signal to distinguish these returns from noise and canopy. This sets the limit on the minimum amount of laser energy that a satellite must emit to allow an accurate measurement, which in turn sets the coverage that a lidar satellite can achieve. Because of the energy requirements, the highest coverage satellite lidar will only directly image around 2-4% of the Earths surface within a 3 year mission. The UK Space Agency funded Global Lidar Altimetry MISsion (GLAMIS) project has been investigating how signal processing, photonics and development in small-sats could reduce the energy requirements for an accurate measurement and so increase the coverage of satellite lidar. Increased coverage would open up many new applications; particularly precise biomass change estimation and global flood modelling.
Maria Valdes Hernandez: Voxel-based statistics of brain imaging data
Abstract
CfS Annual Conference 2022
JCMB, King's Buildings
Invited Talks in Lecture Theatre A
Coffee, Lunch and Posters in Teaching Studio 3217